moabb.paradigms.motor_imagery.SinglePass#
- class moabb.paradigms.motor_imagery.SinglePass(fmin=8, fmax=32, **kwargs)[source]#
Single Bandpass filter motor Imagery.
Motor imagery paradigm with only one bandpass filter (default 8 to 32 Hz)
- Parameters
fmin (float (default 8)) – cutoff frequency (Hz) for the high pass filter
fmax (float (default 32)) – cutoff frequency (Hz) for the low pass filter
events (List of str | None (default None)) – event to use for epoching. If None, default to all events defined in the dataset.
tmin (float (default 0.0)) – Start time (in second) of the epoch, relative to the dataset specific task interval e.g. tmin = 1 would mean the epoch will start 1 second after the beginning of the task as defined by the dataset.
tmax (float | None, (default None)) – End time (in second) of the epoch, relative to the beginning of the dataset specific task interval. tmax = 5 would mean the epoch will end 5 second after the beginning of the task as defined in the dataset. If None, use the dataset value.
baseline (None | tuple of length 2) – The time interval to consider as “baseline” when applying baseline correction. If None, do not apply baseline correction. If a tuple (a, b), the interval is between a and b (in seconds), including the endpoints. Correction is applied by computing the mean of the baseline period and subtracting it from the data (see mne.Epochs)
channels (list of str | None (default None)) – list of channel to select. If None, use all EEG channels available in the dataset.
resample (float | None (default None)) – If not None, resample the eeg data with the sampling rate provided.
Examples using moabb.paradigms.motor_imagery.SinglePass
#
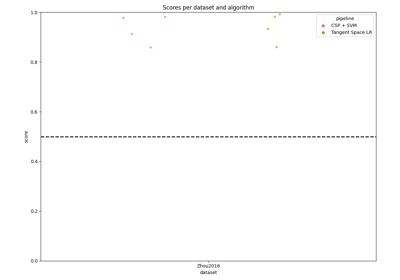
Examples of how to use MOABB to benchmark pipelines.
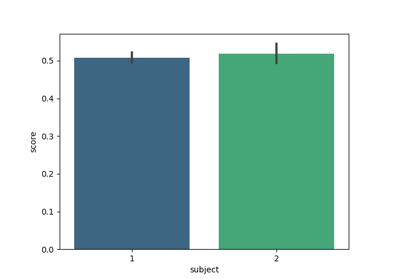
Cross-session motor imagery with deep learning EEGNet v4 model